Blog
Overcoming One Size Fits All Learning
By Guest Blogger, Will Thalheimer, PhD/ @willworklearn When we build e-learning, our goal is to help our learners learn and perform better. But what ...

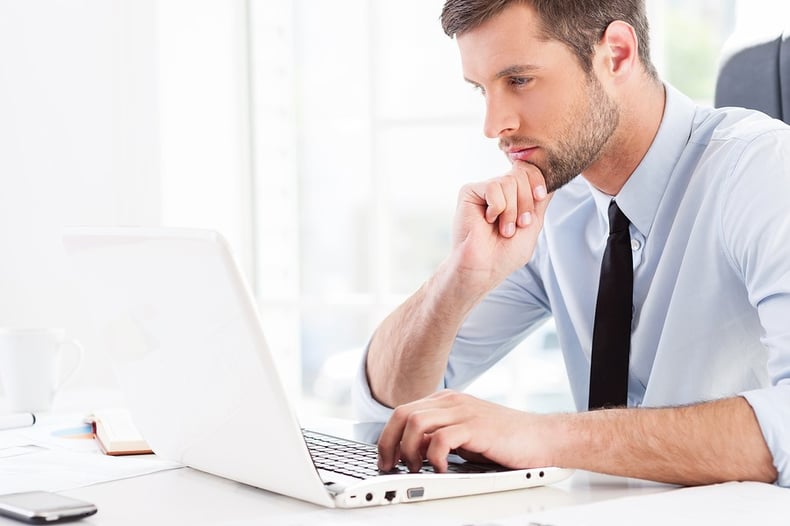
E-Learning Measurement: Creating an eCycle of Continuous Improvement
By Will Thalheimer | March 03, 2016 | Custom Learning | 0 Comments
By Guest Blogger, Will Thalheimer, PhD/ @willworklearn
When we build e-learning, our goal is to help our learners learn and perform better. But what about us as e-learning professionals, shouldn’t we be learning too? Shouldn’t we learn how to perform better in our roles as creators of learning? We should. Obviously! But we don’t! Hardly any of us utilize e-learning’s inherent capabilities to give ourselves feedback on the success/failure of our e-learning.
Hang your head down low!
Seriously! Baseball players get feedback every time they come to the plate. They see how well they hit the ball. They make adjustments. They strive to improve. Product managers do the same thing. They develop a marketing campaign aligned with their brand. They see how effective the campaign is. They make adjustments. Carpenters utilize a new type of clapboard. They learn how well it cuts, how easy it is to work with, how well it takes paint. They make adjustments. Customer service agents try out a new response when faced with a complaint. They see how well the response is received. They make adjustments.
Do we do the same thing as e-learning developers? Do we get good feedback on our e-learning? Do we make adjustments? Do we make improvements?
Even more crucially, do we as instructional developers utilize work processes that incorporate feedback loops and cycles of continuous improvement? Or do we just fling our e-learning newspapers onto the front porches of our learners and assume that they use the content and improve their performance?
Raise your hand if you’re feeling a little sheepish!
Feedback’s Primacy in Human Affairs
The notion of feedback is so critical, so elemental to human existence, that we may not even notice its central importance to our lives and our work. Babies thrive only when their parents observe closely and reflect back at them with warm and meaningful feedback. Infants learn language by listening to the words that surround them and noticing what follows what.
Even in the gray world of the organization, feedback is known as a killer app. Here’s what some notables have said:
- Bill Gates:
“We all need people who will give us feedback. That's how we improve.”
- Elon Musk:
- Ken Blanchard:
“Feedback is the breakfast of champions.”
Beyond the world of work, feedback is also key:
- Barbara Tuchman:
“Learning from experience is a faculty almost never practiced.”
- Helen Keller:
“Character cannot be developed in ease and quiet. Only through experience of trial and suffering can the soul be strengthened, ambition inspired, and success achieved."
Even in the world of instructional design, the wisest among us recognize the importance of feedback. The ADDIE model’s “E” connotes Evaluation to support improvement, though it doesn’t always work that way in practice. Michael Allen’s book with Richard Sites—Leaving ADDIE for SAM—has as its central nugget the primacy of feedback. They argue persuasively that e-learning development is radically improved when we seek feedback and seek it fast—at every step in the development process. Feedback not as an annual checkup, but as the beating heart of learning development! Julie Dirksen amplifies this in her work on Guerrilla Evaluation, where she stresses the importance of feedback loops.
Missing Feedback Loops
Some of you may want evidence of my claim that we are not doing all that we can to get good feedback. Fair enough. Here’s what I’ve seen that we’re missing:
-
We don’t see our learners using our e-learning.
-
Our e-learning smile sheets are either non-existent or ineffective.
-
Our tests of learner understanding focus on low-level content rather than meaningful decision-making.
-
Our tests of learner understanding completely ignore the imperative to measure our learners’ ability to remember.
-
Our attempts to measure on-the-job performance are usually non-existent, and when they’re done at all, they use poorly-conceived subjective opinions of learners.
Lack of User Testing
Our compatriots who facilitate classroom learning get feedback every time they teach. They see how their learners react. Certainly, classroom training doesn’t always provide good feedback. Some instructors are better than others in monitoring their learners—and some instructional designs are better at surfacing levels of learner competence than others.
Unfortunately, most learning developers never see their e-learning programs in use. I learned about this oversight from Julie Dirksen—author of the wonderful Design for How People Learn—who regularly asks her workshop participants whether they’ve EVER watched their learners actually use their online courses. As Julie reported to me, a majority of folks never see learners use their final instructional products. I’ve started asking the same question in my conference sessions, and found the same thing. Most of us just post our e-learning courses to our LMSs—never seeing how our learners experience the learning. I suppose we just assume that all is well.
As Julie has said, this is not only unconscionable and short-sighted, but today’s online-meeting software (GoToMeeting, Webex, etc.) makes it very easy to watch our learners as they engage with our online creations.
I once ran a focus group for a client where the company’s head of e-learning sat at the back of the room, more or less playing the role of my assistant. None of the participants knew she was in charge. The focus group looked at many aspects of training at this large corporation. What the employees hated the most was the e-learning. They couldn’t stop disparaging it. The head of e-learning was stunned. She had seen smile sheet data and knew there were a few complaints, but she just had no idea how bad it was. User testing would have made things clear!
E-Learning has another opportunity in terms of capturing user data. We can very easily keep track of learner clicks, mouse movements, time on-screen, pathways chosen, etc. When I do e-learning audits, I often encounter situations where it would be very helpful to know how long learners spent engaging with a particular screen or exercise—yet alas, in almost every case, the e-learning program was not designed to capture this very simple data.
Poor Smile Sheets
Smile sheets—questions we use to get learners to rate different aspects of our learning interventions—are sometimes overlooked in e-learning. We often just don’t provide such questions. Despite their deficiencies, smile-sheet-type questions can provide an early-warning signal. Even if you only use a few questions, you’ll probably get some valuable information. But you’ve got to be careful!
Too many smile-sheet questions are simply not effective in helping us assess learning. In fact, two meta-analyses, looking at more than 150 scientific studies, found virtually no correlation between traditional smile sheets and learning outcomes. In my book, Performance-Focused Smile Sheets: A Radical Rethinking of a Dangerous Art Form, I go into this research in more depth. The bottom line is that we can’t continue to use poorly-designed smile-sheet questions and expect to get good feedback.
One of our beliefs about learners and learning turns out to be only partially true. We’d like to believe that our learners know how to learn best, but it turns out that they regularly make poor decisions about their own learning (see Brown, Roediger & McDaniel, 2014; Kirschner & van Merriënboer, 2013). Given this blind spot, it’s not enough just to ask learners questions. We have to ask them questions that they can be good at answering.
In brief, here are my recommendations for designing smile-sheet questions (taken directly from the book):
-
Remind learners of the content and the learning experience to ensure they consider the full learning experience.
-
Persuade learners to attend to smile sheets with full engagement.
-
Ensure smile sheets are not too long and are not too short.
-
Follow up with learners to let them know that the smile-sheet results were reviewed and utilized in redesign.
-
Use descriptive answer choices. Do not use numbers, fuzzy adjectives, or other vague wording.
-
Avoid using affirmations in your questions.
-
Use answer choices that have a balance between positive and negative responses.
-
Use answer choices that offer the full range of choices that might be expected.
-
Seek a measurement expert to check your question wording to help you avoid biases and unclear language.
-
Never ask a question that you can’t or won’t be able to use to make an improvement.
-
Don’t ask learners questions that they won’t be good at answering.
-
Balance the length and precision of your answer choices—keeping the answers as short as possible, but lengthening them as needed to make them precise enough so that learners know what they mean.
-
Provide delayed smile sheets in addition to in-the-learning smile sheets.
-
Do not transform smile-sheet results into numbers.
-
Delineate standards of success and failure—acceptable results versus unacceptable results—in advance of training.
-
Ensure your smile sheets measure factors that are aligned with science-of-learning recommendations, for example, the four pillars of training effectiveness: (1) understanding, (2) remembering, (3) motivation to apply, and (4) after-training supports.
-
Design your smile sheets as opportunities to send stealth messages—messages that hint at good learning-design practices.
Poor Measures of Learner Comprehension
As outlined in the research-to-practice report, Measuring Learning Results: Creating Fair and Valid Assessments by Considering Findings from Fundamental Learning Research, there are several biases in the way we typically measure learning:
1. One of the biggest problems is that we tend to use questions in our tests of learning that focus on trivial information or low-level content. Sharon Shrock and Bill Coscarelli, in their classic text, Criterion-Referenced Test Development, call these memorization questions. In the third edition of their book—the current edition—they state that memorization questions are NOT a good indicator of learning—and they recommend against their use. They say specifically that it’s only fair to judge someone as competent in a particular skill by (1) asking them to perform a real-world task, (2) asking them to perform in a well-designed simulation, or (3) asking them to make well-crafted scenario-based decisions. Unfortunately, most e-learning knowledge checks utilize memorization questions. This is particularly troubling because scenario-based questions are relatively easy to write and deploy. Of course, there are some important considerations, namely figuring out how to overcome the weaknesses of the multiple-choice format, but with a little guidance, scenario-based questions can provide powerful nuggets for learning measurement and learning design.
2. The second bias we succumb to in learning measurement is measurement timing. We most often test learners immediately at the end of learning—when information is top-of-mind. While such timing enables us to get a sense of how much learners understand at that point in time, it fails to give us any indication about whether learners will remember and apply what they’ve learned. We need delayed tests for that. Fortunately, e-learning offers an opportunity in this regard. By spreading learning over time through a subscription-learning model (see SubscriptionLearning.com), we can provide delayed assessment questions that won’t actually feel like assessment questions to our learners. To them, they are just another realistic decision to answer. To us, we can use the results to get feedback on the success of our e-learning to support remembering.
3. The third bias common in learning measurement is measuring learners while they are still in their learning situations. It turns out that when we learn something in one situation, we can remember that information better in that situation than in other situations. This is a bigger problem for classroom training, because classrooms are clearly not like people’s work stations. For e-learning, learners are often sitting at their desks. But still, where e-learning provides cues more salient than the workplace environment—from design elements within the e-learning—we may still be creating hints that won’t be available when the e-learning is not present.
To summarize this section, in our e-learning we ought to measure learners (1) utilizing realistic scenario-based decisions or simulations, (2) both during learning and after a substantial delay, and (3) in a manner that doesn’t provide contextual hints—unless those hints are the same ones learners will have when the e-learning is not present.
Poor Measures of On-the-Job Application
Rarely do we measure actual job performance as a result of our e-learning. When we do, we tend to use poor smile-sheet-like questions sent to learners after the learning event. Many people will even call these results Level 3 results (from the Kirkpatrick four-level model), attempting to indicate that they are capturing job-performance data. While I’m sympathetic to the need for trying to measure performance, questioning learners is a weak method in this regard—made worse because of the poor questions we typically use.
Obviously, it’s better to use more direct measures of job behavior (for example, level of sales), customer satisfaction data, etc. When this is logistically impossible or too difficult, if we are going to ask questions of learners, we ought to follow some of the same recommendations we’d use to improve our smile sheets.
The good news on the horizon is that the future of e-learning measurement will include better performance data, enabled through tools such as: xAPI, which allows learning AND job-performance data to be cataloged and stored; HPML (Human Performance Measurement Language), which will facilitate this process; and mobile-learning apps like Marty Rosenheck’s TREK system, which supports virtual coaching and feedback, and can provide more data to mine and analyze to find improvement opportunities.
Summing It Up
E-Learning provides a powerful tool to support learners in learning. It also has—inherent in its design—the ability to capture data—data that can give us feedback. Moreover, e-learning has the capability to be spaced over time, enabling us to gain insight into whether our e-learning designs are supporting our learners in remembering.
In the Serious eLearning Manifesto—created by Michael Allen, Julie Dirksen, Clark Quinn, and me—only one of the 22 principles goes beyond a few sentences. Only one e-learning-design meme is important enough to clarify with multiple specifications: Learning measurement.
I might preach redemption and righteousness and our professional responsibility to create virtuous cycles of continuous improvement in e-learning. But who wants to hear that!
Why might you want to improve your e-learning measurement? First, it’s relatively easy. Second, you’ll better help your learners. Third, you’ll probably feel better about what you do—seeing your efforts visibly improve in effectiveness. Fourth, you might make your job more secure. By creating more effective e-learning, you might avoid being tossed to the side when times get tough. Fifth, better e-learning can help create a competitive advantage for your company. Sixth, and this will be the last one, it is fun to do good learning measurement! In my book, I say, “Learning measurement is pure sex.” Really! I do say that. This isn’t just a shameless plug; it’s the truth! SMILE…
Author Bio
Will Thalheimer, PhD, is a consultant and research translator, providing organizations with learning audits, research benchmarking, workshops, and strategic guidance. Will shares his wisdom through keynotes, research reports, job aids, workshops, and blog posts. Compiler of the Decisive Dozen, one of the authors of the Serious eLearning Manifesto (eLearningManifesto.org), founder of The Debunker Club (Debunker.Club), and author of the highly-acclaimed book Performance-Focused Smile Sheets (SmileSheets.com), Will blogs at WillAtWorkLearning.com, tweets as @WillWorkLearn, and consults through Work-Learning Research, Inc. (Work-Learning.com). Will regularly publishes extensive research-to-practice reports—and gives them away for free.
Citations
Allen, M.W., & Sites, R.H. (2012). Leaving ADDIE for SAM: An Agile Model for Developing the Best Learning Experiences. Alexandria, VA: ASTD Press. Available at: http://www.amazon.com/Leaving-Addie-Sam-Developing-Experiences/dp/1562867113
Alliger, G. M., Tannenbaum, S. I., Bennett, W., Jr., Traver, H., & Shotland, A. (1997). A meta-analysis of the relations among training criteria. Personnel Psychology, 50, 341–358.
Brown, P. C., Roediger, H. L., III, & McDaniel, M. A. (2014). Make It Stick: The Science of Successful Learning. Cambridge, MA: Belknap Press of Harvard University Press.
Dirksen, J. (2015). Design for How People Learn (Voices that Matter). Second Edition. New Riders. Available at: http://www.amazon.com/Design-People-Learn-Voices-Matter/dp/0134211286/
Kirschner, P. A., & van Merriënboer, J. J. G. (2013). Do learners really know best? Urban legends in education. Educational Psychologist, 48(3), 169–183.
Shrock, S. A., & Coscarelli, W. C. (2007). Criterion-Referenced Test Development: Technical and Legal Guidelines for Corporate Training, 3rd ed. San Francisco: Wiley. Available at: http://www.amazon.com/Criterion-referenced-Test-Development-Technical-Guidelines/dp/1118943406/
Sitzmann, T., Brown, K. G., Casper, W. J., Ely, K., & Zimmerman, R. D. (2008). A review and meta-analysis of the nomological network of trainee reactions. Journal of Applied Psychology, 93(2), 280–295.
Thalheimer, W. (2016). Performance-Focused Smile Sheets: A Radical Rethinking of a Dangerous Art Form. Somerville, MA: Work-Learning Press. Available at: SmileSheets.com
Thalheimer, W. (2007, April). Measuring Learning Results: Creating fair and valid assessments by considering findings from fundamental learning research. Available at http://Work-Learning.com/Catalog.html.
LIKE WHAT YOU'VE READ? SHARE THE KNOWLEDGE WITH YOUR PEERS USING THIS READY-MADE TWEET!
CLICK TO TWEET: #eLearning Measurement: Creating an #eCycle of Continuous Improvement http://hubs.ly/H02gfFQ0 #aiblog by @willworklearn
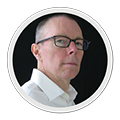
About the Author: Will Thalheimer
Comments
Would you like to leave a comment?
Related Blog Posts
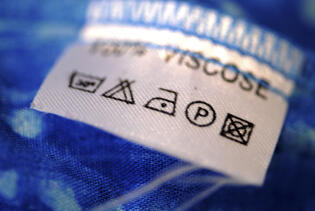
By: Will Thalheimer | Nov, 2014
Category: Custom Learning
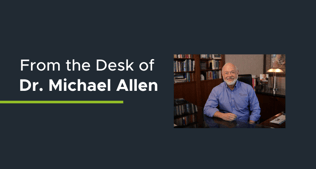
Blog
Today's eLearning: Michael Allen & Experts Say "Enough is Enough"
By Guest Blogger, Will Thalheimer, PhD/ @willworklearn When we build e-learning, our goal is to help our learners learn and perform better. But what ...
By: Will Thalheimer | Mar, 2014
Category: Custom Learning, Strategic Consulting, Dr. Michael Allen
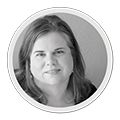
Blog
An e-Learning Challenge – Why Should You Care Right Now?
By Guest Blogger, Will Thalheimer, PhD/ @willworklearn When we build e-learning, our goal is to help our learners learn and perform better. But what ...
By: Will Thalheimer | Nov, 2013
Category: Custom Learning